HOLISMOKES XVI: Lens search in HSC-PDR3 with a neural network committee and post-processing for false-positive removal
We have carried out a systematic search for galaxy-scale lenses exploiting multi-band imaging data from the third public data release of the Hyper Suprime-Cam (HSC) survey with the focus on false-positive removal, after applying deep learning classifiers to all ∼110 million sources with i-Kron radius above 0.8". To improve the performance, we tested the combination of multiple networks from our previous lens search projects and found the best performance by averaging the scores from five of our networks. Although this ensemble network leads already to a false-positive rate (FPR) of ∼0.01% at a true-positive rate (TPR) of 75% on known real lenses, we have elaborated techniques to further clean the network candidate list before visual inspection. In detail, we tested the rejection using SExtractor and the modeling network from HOLISMOKES IX, which resulted together in a candidate rejection of 29% without lowering the TPR. After the initial visual inspection stage to remove obvious non-lenses, 3,408 lens candidates of the∼110 million parent sample remained. We carried out a comprehensive multi-stage visual inspection involving eight individuals and identified finally 95 grade A (average grade G ≥2.5) and 503 grade B (2.5 >G ≥1.5) lens candidates, including 92 discoveries showing clear lensing features that are reported for the first time. This inspection also incorporated a novel environmental characterization using histograms of photometric redshifts. We publicly release the average grades, mass model predictions, and environment characterization of all visually inspected candidates, while including references for previously discovered systems, which makes this catalog one of the largest compilation of known lenses. The results demonstrate that (1) the combination of multiple networks enhances the selection performance and (2) both automated masking tools as well as modeling networks, which can be easily applied to hundreds of thousands of network candidates expected in the near future of wide-field imaging surveys, help reduce the number of false positives that is the main limitation in lens search to date.
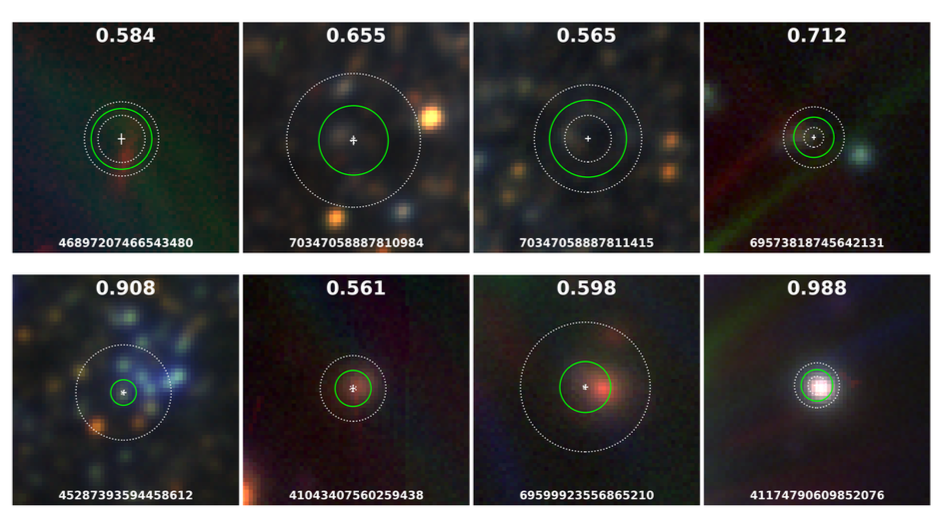
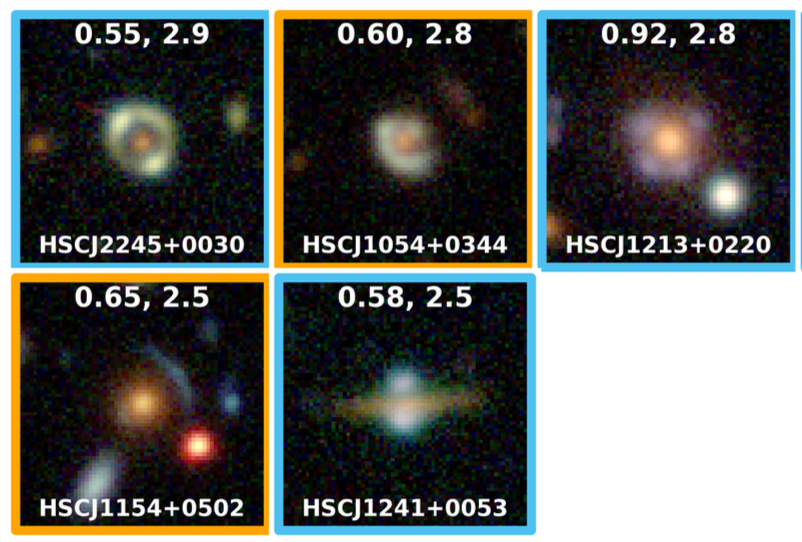