HOLISMOKES. XIII. Strong-lens candidates at all mass scales and their environments from the Hyper-Suprime Cam and deep learning
We have performed a systematic search for galaxy-scale strong gravitational lenses using Hyper Suprime-Cam (HSC) imaging data, focusing on lenses in overdense environments. To identify these lens candidates, we exploit our residual neural network from HOLISMOKES VI, which is trained on realistic gri mock-images as positive examples, and real HSC images as negative examples. Compared to our previous work, where we have successfully applied the classifier to around 62.5 million galaxies having i-Kron radius ≥ 0.8", we now lower the i-Kron radius limit to ≥ 0.5". This results in an increase by around 73 million sources to more than 135 million images. During our visual multi-stage grading of the network candidates, we now also inspect simultaneously larger stamps (80" × 80") to identify large, extended arcs cropped in the 10" × 10" cutouts and classify additionally their overall environ- ment. Here, we also re-inspect our previous lens candidates with i-Kron radii ≥ 0.8" and classify their environment. Using these 546 visually identified lens candidates, we further define various criteria by exploiting extensive and complementary photometric redshift catalogs, to select the candidates in overdensities. In total, we identified 24 grade-A and 138 grade-B candidates with either spatially- resolved multiple images or extended, distorted arcs in the new sample. Furthermore, combining our different techniques, we identify in total 237/546 lens candidates in a cluster-like or significant overdense environment, containing only 49 group- or cluster-scale re- discoveries. These results demonstrate the feasibility of downloading and applying neural network classifiers to hundreds of million cutouts, necessary in the upcoming era of big data from deep, wide-field imaging surveys like Euclid and the Rubin Observatory Legacy Survey of Space and Time, while leading to a sample size that can be visually inspected by humans. These deep learning pipelines, with false-positive rates of ∼ 0.01%, are very powerful tools to identify such rare galaxy-scale strong lensing systems, while also aiding in the discovery of new strong lensing clusters.
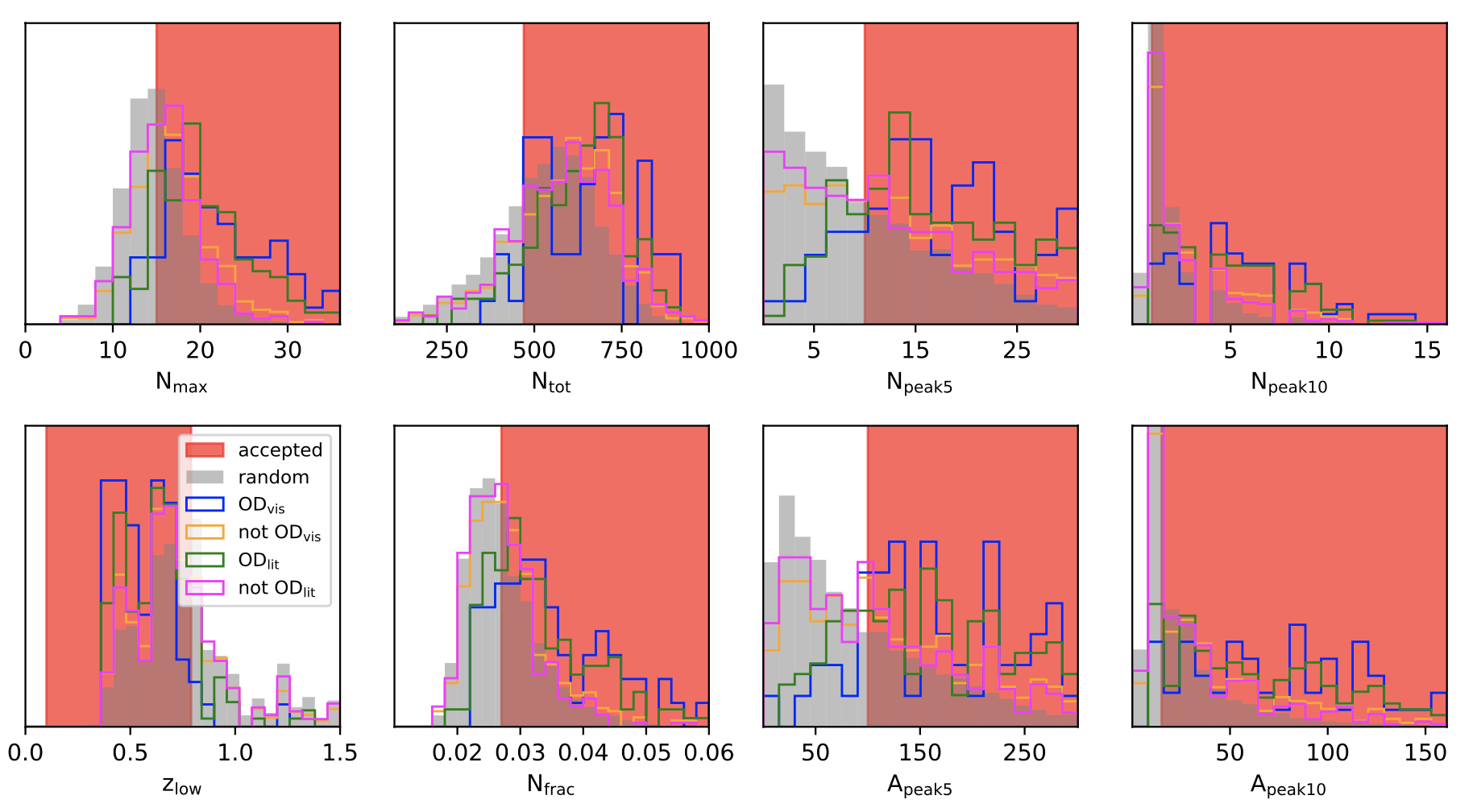