HOLISMOKES. VI. New galaxy-scale strong lens candidates from the HSC-SSP imaging survey
Expanding the current samples of galaxy-scale strong lenses is important for galaxy evolution and cosmology. These systems provide not only valuable diagnostics on the foreground lens mass distributions, but also independent and competitive measurements of the Hubble constant H0 when the background sources exhibit time variations. In particular, finding strongly lensed supernovae will become routinely possible with the Rubin Observatory Legacy Survey of Space and Time (LSST), and their analysis for H0 inference will require rapid identification and follow-up. The successful analysis of strongly lensed supernovae will therefore require an automated selection of large samples of static strong lenses from the early LSST stacks.
In H0LISMOKES II, we show that realistic lens simulations and supervised classification with convolutional neural networks can efficiently select strong lenses over 30,000 deg2 from PanSTARRS multi-band imaging. Here, we further develop supervised neural networks for automated selection of strong lenses in large-scale multiband surveys approaching the expected data quality of LSST. In particular, we focus on systems with wide image separations (Einstein radii θE ~ 1.0–3.0"), intermediate redshift lenses (z ~ 0.4–0.7), and bright arcs. We develop and test our pipelines on images from the Wide layer of Hyper-Suprime Cam Subaru Strategic Program (HSC-SSP; Aihara et al. 2018), which are only ~1 mag shallower than LSST ten-year stacks. This allows us to carefully test the classification purity and completeness as a function of the ground truth data sets and network architectures, using a test set closely representative of the actual HSC sample for lens search. We find that using the most realistic lens simulations and deep Residual Networks can perform a lens selection with 0.01% false positive rate and >50% completeness on previously published lens systems. Such a low contamination enables one to identify strong lenses over an extended galaxy sample without strict catalog-level preselections, and with a limited human inspection time.
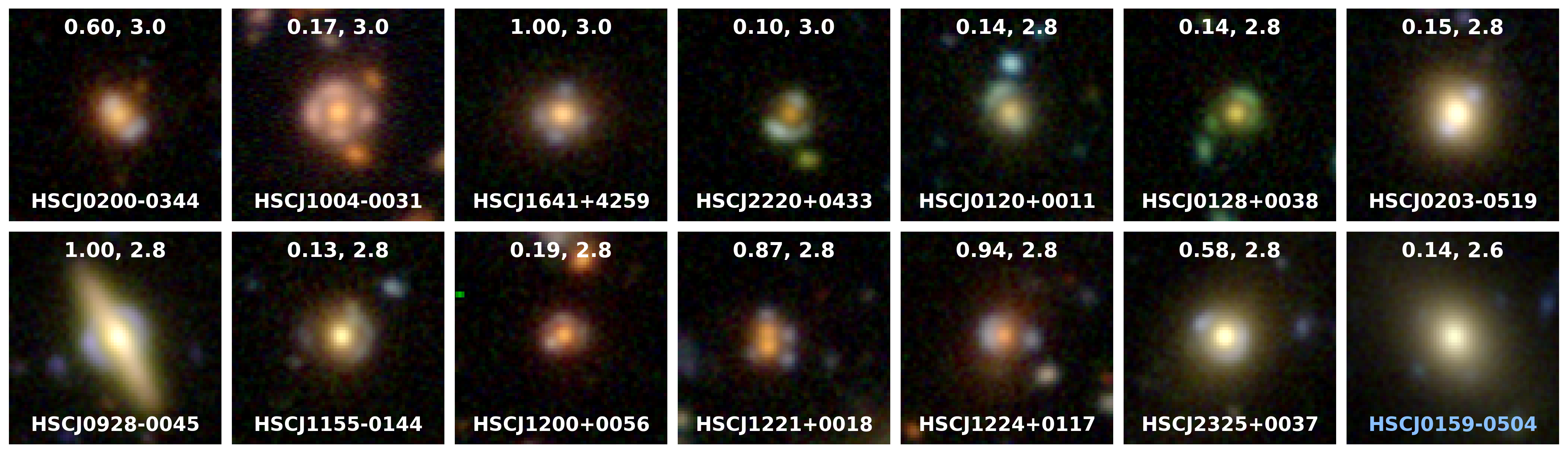
We apply our pipeline to the 62.5 million galaxies with i-band Kron radius ≥0.8" in DR2 of HSC-SSP Wide. The classification performance closely matches expectations from our test set. By reducing the contamination in the sample of neural network candidates, we can rapidly collect 206 newly-discovered definite or probable lenses with either spatially-resolved multiple images or extended, distorted arcs (see examples in the figure). In addition, we find 88 high-quality candidates that were assigned lower confidence in previous HSC searches, and we recover 173 known systems in the literature. These results show that our pipeline is adjustable to LSST and can largely extend the samples found by traditional non machine learning algorithms. We finally provide a ranked list of candidates for future spectroscopic confirmation.