HOLISMOKES. XII. Time-delay Measurements of Strongly Lensed Type Ia Supernovae using a Long Short-Term Memory Network
Strongly lensed Type Ia supernovae (LSNe Ia) are a promising probe to measure the Hubble constant (H0) directly. To use LSNe Ia for cosmography, a time-delay measurement between the multiple images, a lens-mass model, and a mass reconstruction along the line of sight are required. In this work, we present the machine learning network LSTM-FCNN which is a combination of a Long Short-Term Memory Network (LSTM) and a fully-connected neural network (FCNN). The LSTM-FCNN is designed to measure time delays on a sample of LSNe Ia spanning a broad range of properties, which we expect to find with the upcoming Rubin Observatory Legacy Survey of Space and Time (LSST) and for which follow-up observations are planned. With follow-up observations in i band (cadence of one to three days with a single-epoch 5σ depth of 24.5 mag), we reach a bias-free delay measurement with a precision around 0.7 days over a large sample of LSNe Ia. The LSTM-FCNN is far more general than previous machine learning approaches such as the Random Forest (RF), where a RF has to be trained for each observational pattern separately, and yet the LSTM-FCNN outperforms the RF by a factor of roughly three. Therefore, the LSTM-FCNN is a very promising approach to achieve robust time delays in LSNe Ia, which is important for a precise and accurate constraint on H0.
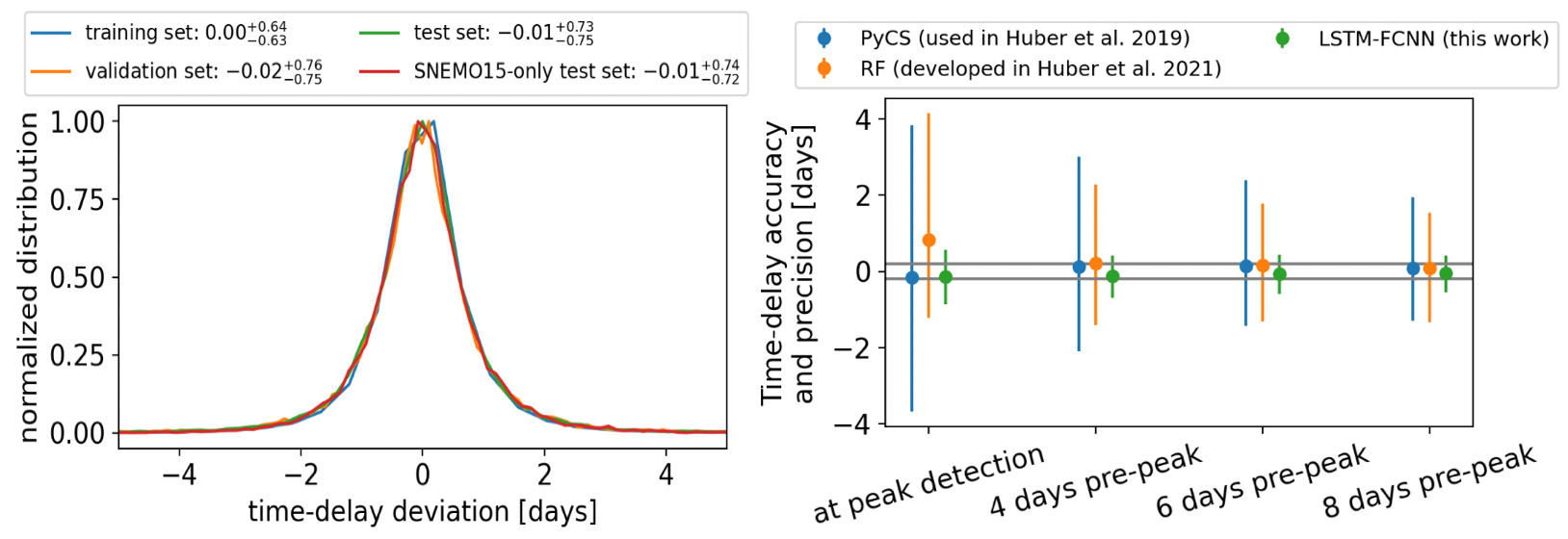