HOLISMOKES. VIII. High-redshift, strong-lens search in the Hyper Suprime-Cam Subaru Strategic Program
The total mass (dark matter + baryonic matter) is arguably the single most important property of a galaxy, and its distribution across the galaxy contains imprints from various key physical processes that have played a role in the formation and evolution of the galaxy. Observationally determining the total-mass distribution of galaxies and its redshift dependence can thus provide a compelling test on the complex baryonic physics, dark matter-baryon interplay, and galaxy evolution models. As a pure gravity-dependent effect, strong gravitational lensing is a powerful technique of measuring the total-mass distribution of galaxies. Studies of strong-lens systems have successfully measured the dark-matter and stellar mass distributions of galaxies at cosmological distances and substantially deepened our understanding of galaxy formation and evolution.
In H0LISMOKES II and H0LISMOKES IV, we searched for strong-lens systems with wide image separations in the PanSTARRS and HSC data, most of which contain lens galaxies at intermediate redshifts (z~0.5). In this paper, we focused on discovering higher-redshift strong lenses from the HSC data, which are severely under-represented in the current strong-lens population.
We constructed two strong-lens classifiers based on a deep residual network developed by Lanusse et al. (2018). The main difference between the two classifiers was on the training set. As a result, we were able to investigate the impact of the training set on the classifier performance. Applying the two classifiers to imaging data from the second public data release of the HSC-SSP, we identified 735 grade-A or B strong-lens candidates in total (see examples below), of which 277 were discovered for the first time. This is the single largest set of galaxy-scale strong-lens candidates discovered with HSC data to date, and nearly half of it contains lens galaxies with photometric redshifts above 0.6.
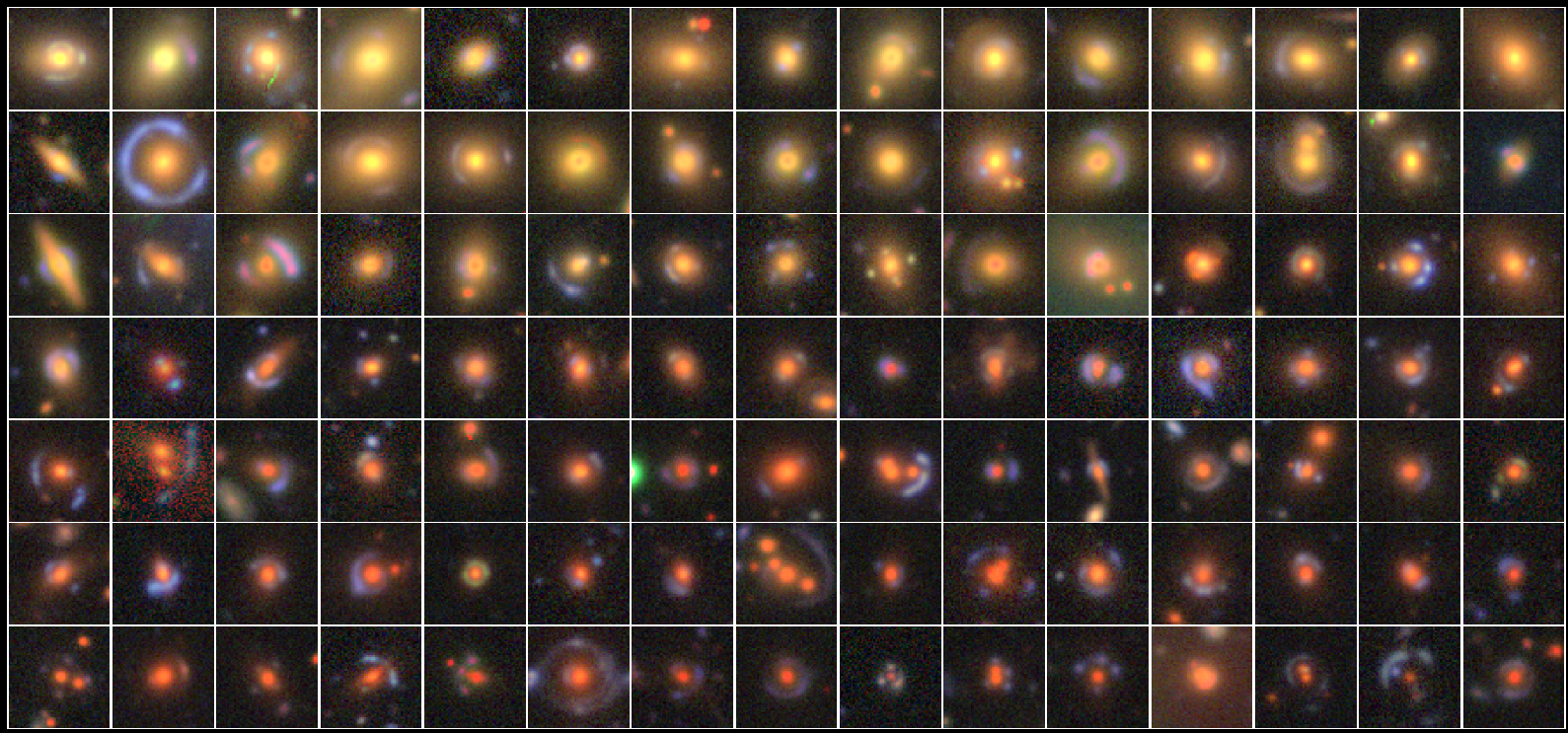
Using a test set selected in the HSC footprint, we examined the performance of our two classifiers. At a false positive rate (FPR) of 0.001, Classifier-1 and Classifier-2 achieved an overall true positive rate (TPR) of 85% and 60% respectively. Breaking down into individual redshift intervals, we found that, although the overall TPR of Classifier-2 is lower than that of Classifier-1, Classifier-2 outperformed Classifier-1 in discovering strong-lens candidates with lens galaxy redshifts above 0.7 (see the figure below). This is understandable because the training set for Classifier-2 contains a significantly higher fraction of z > 0.7 strong-lens sytems. It highlights that the outcome of supervised machine learning techniques depends strongly on the training set.
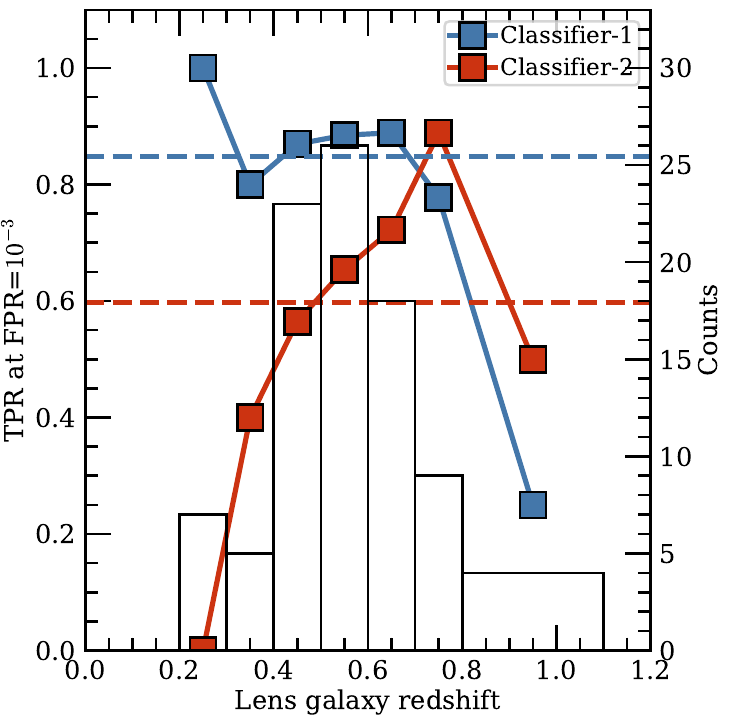