HOLISMOKES. II. Identifying galaxy-scale strong gravitational lenses in Pan-STARRS using convolutional neural networks
Strongly lensed supernovae with wide image separations are particularly promising for cosmography and for supernova progenitor studies. Their longer time delays of a few days to weeks between multiple images are relatively less sensitive to microlensing effects, and make it easier to trigger timely imaging and spectroscopic follow-up. One way to select these systems is to expand current samples of galaxy-scale strong gravitational lenses and wait for a supernova to explode in one of the background lensed galaxies. For that purpose, we performed a systematic machine learning search for galaxy-scale strong lenses in the Pan-STARRS 3π imaging survey over the entire Northern extragalactic sky. We focused our search on massive luminous red galaxies acting as strong lenses and producing large Einstein radii ≥1.5”.
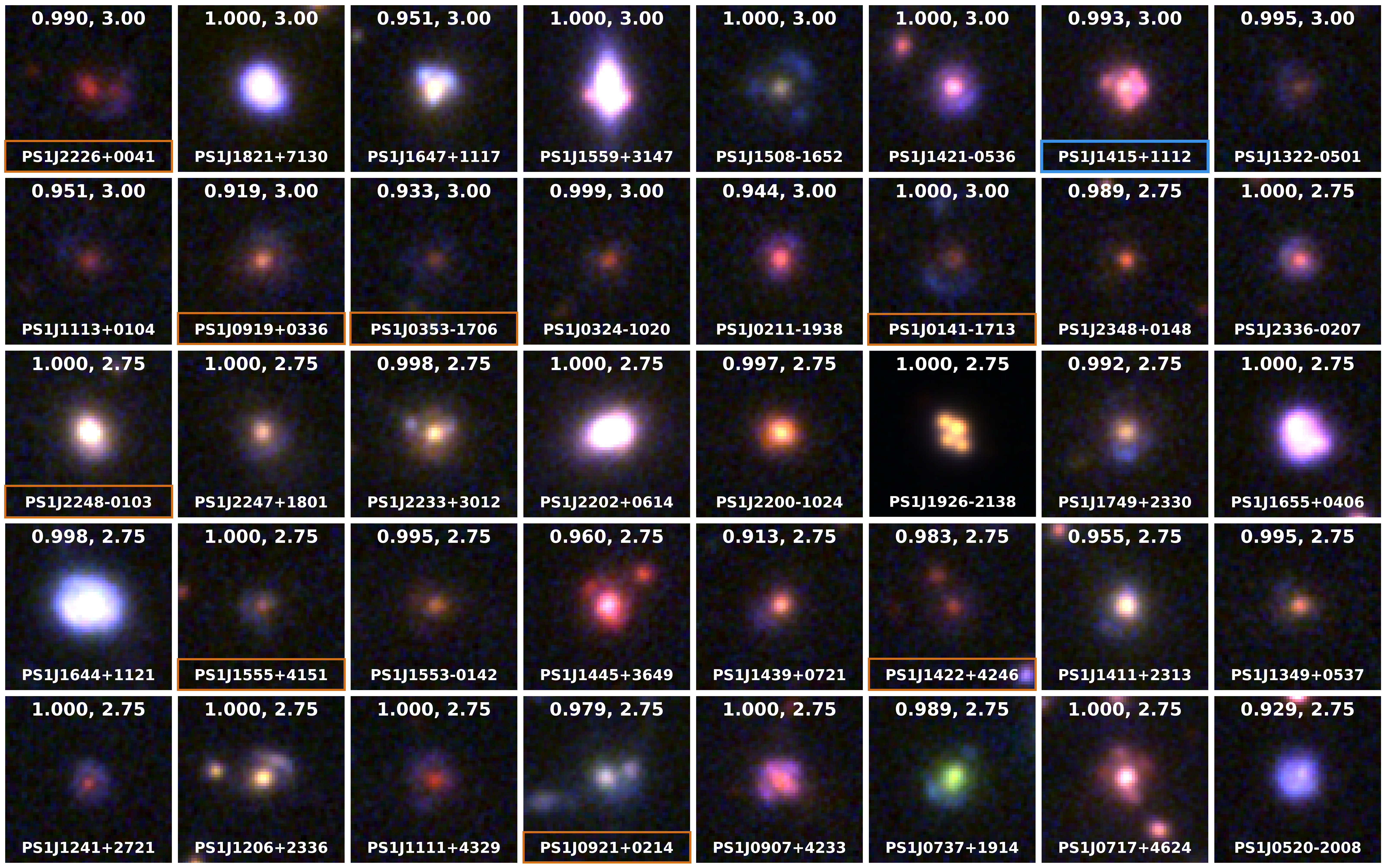
Our CNN yields 12 382 candidates with scores pCNN >0.9, respectively, which we visually inspect to assemble a final set of 330 high-quality newly-discovered lens candidates with average visual grades ≥2. The figure above shows the Pan-STARRS 3-color gri images of a subset of candidates with CNN scores pCNN > 0.9 (top-left of each panel) and with the highest visual grades (top-right). Those with Pan-STARRS names marked in orange have been previously published in the literature. Publicly available BOSS spectroscopy of the central regions of the candidate lens galaxies confirms that the vast majority are indeed luminous red galaxies at z~0.1−0.7. Moreover, five of our newly-discovered candidates show robust spectral signatures of blended, high-redshift background sources that are likely lensed. Pan-STARRS unambiguously confirms one of these five systems (marked in blue in the figure) as a quadruply-imaged red galaxy at zs = 1.185 (likely recently quenched), behind a lens galaxy at zd = 0.3155.
In the future, validating our list of Pan-STARRS lens candidates and deriving strong lensing models will require high-resolution imaging and spectroscopic follow-up. We expect that the efficient and automated two-step classification method presented in this paper will be applicable to the deep gri image stacks from LSST with only minor adjustments.